A Glimpse at 2024’s AI Security Landscape
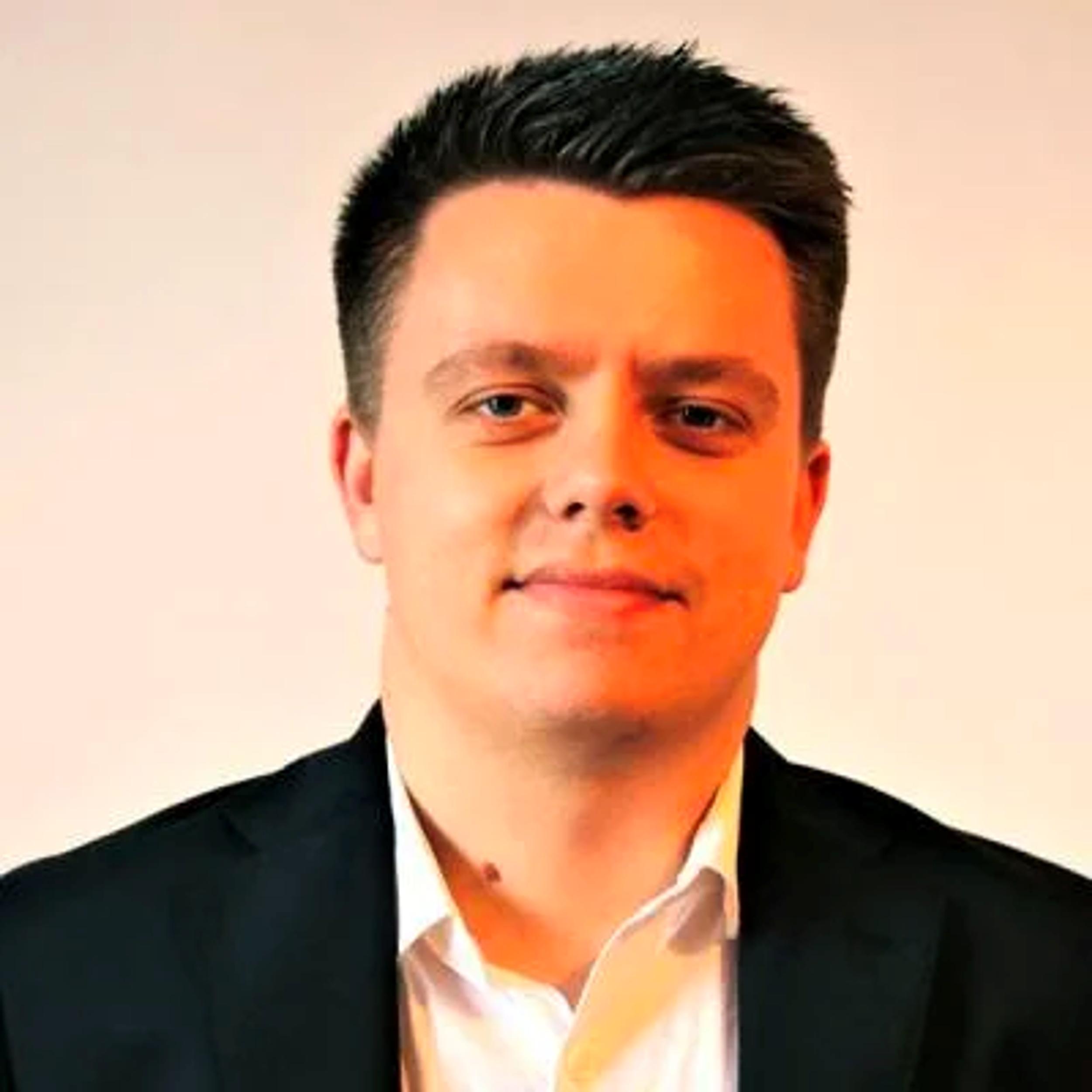
Today’s AI ecosystem is a fast-growing environment of both new applications and new security threats as AI technologies mature at a record-breaking pace. 2023 was a banner year for AI innovation, driven by the widespread adoption of ChatGPT. As well as advances in AI-driven applications that are multiplying across industry sectors and enterprise use cases. Along with this surge of innovation, however, comes expanding attack surfaces and novel cyber threats that specifically target AI systems.
Some of the most impactful work in 2024 will focus on strengthening security and governance for AI efficacy while guarding against increasingly virulent, tailor-made threats to exploit AI technology. As we move into 2024.
Data poisoning is the process of manipulating algorithms so that malicious actors can control their output. As just one example, data poisoning helped attackers tamper with Google’s anti-spam filters to allow their phishing emails to bypass the filter. This technique will continue to grow as bad actors gain access to greater computing power and new tools – as of today. Data poisoning has become the most critical vulnerability in machine learning and AI. The drastic impact of data poisoning places a premium on keeping adversaries away from data and training models as organizations use artificial intelligence and machine learning for a broader range of use cases. Particularly those that involve essential services like healthcare, transportation, and policing. Where a successful attack can potentially have life-threatening impacts.
Especially since OpenAI and Microsoft unleashed ChatGPT in November 2022, AI has been increasingly weaponized to facilitate malicious attacks. As just one example, dark web customers can acquire FraudGPT to conduct human-like interactions with potential victims to carry out fraudulent activities; and large language models (LLMs) can also be used to create sophisticated malware capable of generating phishing attacks and data exfiltration. Making matters worse, the barrier to entry for such attacks is low – especially when attackers opt for homespun methods to train the LLM on plaintext datasets, such as using a standard commodity item like a gaming PC to do the required computation.
The above threats are just a sampling of the many reasons why risk mitigation must evolve in the face of AI, and this evolution must go beyond the instinct to simply prohibit the use of new AI tools. In a world where ChatGPT has now set the record for the fastest-growing user base in history, there’s no going back. That means risk mitigation must evolve to better define, implement, and enforce AI policies. In fact, AI security policies will likely become as essential to defending a business’ competitiveness as confidentiality policies are today. Such policies should focus protections on the advanced nature of the threat.
To guard against data poisoning, for instance, risk mitigation efforts can use statistical methods to detect anomalies and Zero Trust Content Disarm and Reconstruction (CDR) to ensure data being transferred is clean and that only authorized users get access to the training data sets. And while AI-generated malware will enable hackers to scale the work that they do, security professionals can match the threat by scaling their protections with platforms designed to monitor and manage access and protect data anywhere AI is used.
Solutions Architect, Western Europe & UK
Aaron Mulgrew is a Solutions Architect, Western Europe & UK, at Everfox. In this capacity, Aaron works with central government departments in the UK and abroad to secure their systems, as well as alongside critical national infrastructure providers to make sure they aren’t an easy route to compromise. Specialising in cryptocurrency protection, Aaron ensures that cryptocurrency service providers maximize their security potential. Aaron brings years of experience in research, technical sales and cloud infrastructure protection to Everfox.