Investigating the Impacts of Data Poisoning
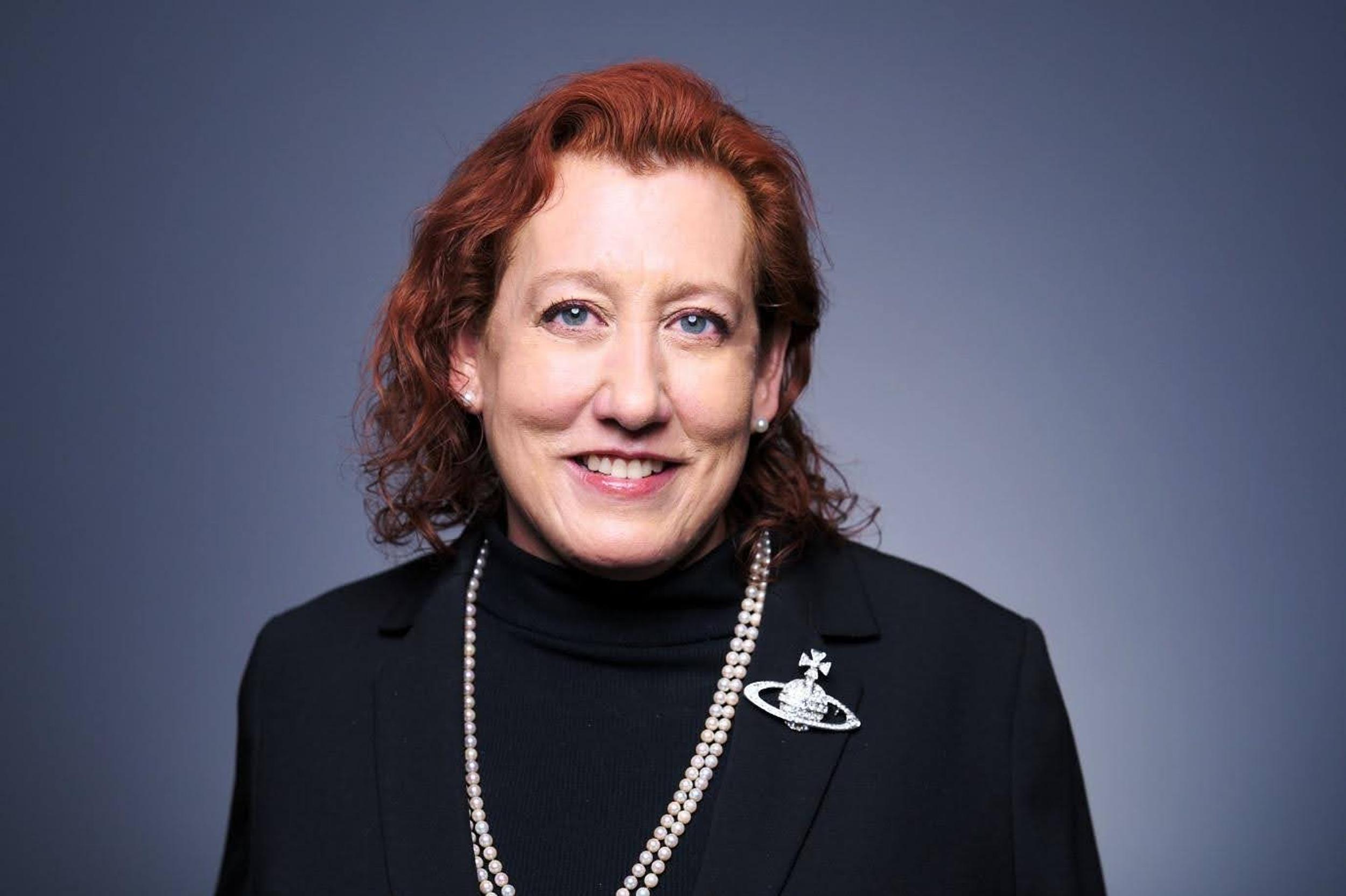
As technology evolves, so do threats.
The public sector is witnessing the integration of machine learning and artificial intelligence, whether government agencies are prepared for it or not.
In the past year, Generative AI has garnered significant attention, with ChatGPT achieving the fastest-growing user base in history. Concurrently, Microsoft introduced a generative AI service tailored for government use in June. The department of Defense further solidified this trend by announcing a generative AI task force in August, and additional initiatives are anticipated to follow suit.
The list of possible use cases for AI is long: It can streamline cumbersome workflows, help agencies more effectively detect fraud and even support law enforcement efforts. Regardless of the application, a consistent truth prevails: This assumes that the data isn’t being edited or added maliciously.
Data poisoning - the manipulation of algorithms through incorrect or compromised data — represents a new threat vector, particularly as more agencies embrace AI. Though not novel, data poisoning attacks have emerged as the most pressing vulnerability in the realm of ML and AI. This shift is attributed to the increased accessibility of bad actors to enhanced computing power and the utilization of new tools.
Data poisoning attacks can be categorized in two ways. 1, by how much knowledge the attacker has and by which tactic they employ. 2, When a bad actor has no knowledge of the data they seek to manipulate, it’s known as a black-box attack.
The other side of the spectrum is a white-box attack, in which the adversary has full knowledge of the model and its training parameters. These attacks, as you might suspect, have the highest success rate.
There are also grey-box attacks, which fall in the middle.
The amount of knowledge a bad actor has may also affect which tactic they choose. Data poisoning attacks, generally speaking, can be broken into four broad buckets: availability attacks, targeted attacks, subpopulation attacks and backdoor attacks. Let’s take a look at each.
Availability attack: With this breed of attack, the entire model is corrupted. As a result, model accuracy will be considerably reduced. The model will offer false positives, false negatives and misclassified test samples. One type of availability attack is label flipping, or adding approved labels to compromised data.
Targeted attack: While an availability attack compromises the whole model, a targeted attack affects only a subset. The model will still perform well for most samples, which makes targeted attacks challenging to detect.
Subpopulation attack: Much like a targeted attack, a subpopulation attack doesn’t affect the whole model. Instead, it influences subsets that have similar features.
Backdoor attack: As the name suggests, this type of attack takes place when an adversary introduces a back door. Such as a set of pixels in the corner of an image — into training examples. This triggers the model to misclassify items.
Within the private sector, Google’s anti-spam filter has faced numerous attacks. Through the manipulation of the spam filter’s algorithm, malicious actors have succeeded in altering the definition of spam, enabling harmful emails to circumvent the filter.
Consider the ramifications if a comparable scenario were to unfold within a government agency. Undoubtedly, the consequences would be considerably more severe.
To start, proactive measures must be put in place. Agencies need to be extremely diligent about which data sets they use to train a given model and who is granted access to them.
When a model is being trained, it’s crucial to keep its operating information secret. This high level of diligence can be enhanced by high speed verifiers and prevention-based content disarm and reconstruction, tools that ensure all data being transferred is clean.
Additionally, statistical models can be used to detect anomalies in the data. While tools such as Microsoft Azure Monitor and Amazon SageMaker can detect shifts in accuracy.
Taking proactive measures is imperative due to the formidable challenge of rectifying data poisoning. Addressing a tainted model requires the agency to undertake a thorough analysis of its training inputs, identifying and eliminating any fraudulent elements.
As data sets grow, that analysis becomes more difficult, if not impossible. In such cases, the only option is to retrain the model completely, a time-consuming and expensive process.
Training GPT-3, for instance, carried a price tag of more than $17 million. Most agencies simply do not have the budget for that kind of correction.
As agencies adopt Machine Learning (ML) and emerging AI technologies, they need to remain vigilant regarding the accompanying threats. Adversaries have various means to disrupt a model, ranging from injecting malicious data to altering existing training samples.
The prevention of data poisoning attacks holds paramount importance, especially as agencies increasingly depend on AI to provide essential services. To unlock the full potential of AI, global agencies must proactively take measures to uphold model integrity across the board.
Senior Director of Global Products at Everfox
Audra is part of the Everfox Global Governments team, where her goal is to break new ground in the area of non-ITAR global products and engineering with a focus on high assurance critical infrastructure customers, markets and solutions. Previously to this role Audra led an Innovation Lab producing cutting-edge technology and service innovations. In all of Audra’s roles she instils a culture of innovation and closely partners with customers, internal leaders and key vendors to drive innovations that solve critical business problems. Prior to this, she has held various roles in global and national services providers driving and delivering innovation and products, rationalisation, and best practices.